Summary: I talked with Brian Sullivan on CNBC’s The Exchange about the impact of China protests on their disruptive Zero-COVID policy. (see a video by clicking here.) But Xi Jinping wasn’t the only autocrat to have a bad week: Vladimir Putin’s family photo was ruined when Armenian Prime Minister Pashinyan got stand-offish; and Belarus President Lukashenko hired a whole new team of cooks and bodyguards after his Foreign Minister died of poison. Finally, I explain why autocrats are bad for investors—the Central Limit Theorem doesn’t work and add an Appendix on Power Law distributions for the statistics geeks among our readers. As always, I welcome your comments.
President Xi is in a tough spot. His signature Zero-COVID policy has locked down more than 400 million people this year and hobbled the Chinese economy. Scores of protests showed that young people are not happy. And former President Jiang Zemin—Xi Jinping’s mentor, who improved relations with the US, brought China into the WTO, secured the 2008 Beijing Olympics, and delivered huge increases in living standards—was inconsiderate enough to die right in the middle of the protests.
Bad Week for Autocrats
It was a bad week for autocrats and that’s a good thing. On Wednesday, Vladimir Putin attended the Collective Security Treaty Organization (CSTO) Summit, to sign a joint security agreement with former USSR Republics Armenia, Belarus, Kazakhstan, Kyrgyzstan and Tajikistan. Armenian Prime Minister Nikol Pashinyan (just to the left of the yellow arrow in the photo) refused to sign the joint CSTO declaration and ruined Putin’s Back in the USSR family photo when he wouldn’t stand next to Vlad. Of course, there could just have been an open window nearby.
Kazakhstan President Tokayev (2nd from left in the photo) made it clear he does not support Russia in Ukraine, that he is leaning toward Europe, and 100,000 Russian draft dodgers are welcome visitors.
Later in the week, the Kremlin released a video of a fake Putin meeting with fake mothers of dead soldiers. The mothers were identified as actors and Putin’s (slightly too large for his body, floating) head was Photoshopped into the picture. Guess the IT guy must have been drafted.
And finally, there were reports that Putin had fallen down the stairs in his residency, injuring his tailbone.
Lukashenko (far left in the photo) had a bad week too. His Foreign Minister suddenly died of poison after Putin learned that Lukashenko was trying to cut a side deal with the Americans and Europeans. Lukashenko replaced his cooks, his bodyguards, and his security staff.
And then there is Xi Jinping, who had a bad week too:
He went from being newly appointed Emperor of China and self-appointed “man of the people” to the butt of mass demonstrations against Zero-Covid, the CCP , the sagging economy, and himself as President-for-life.
Former President Jiang Zemin died while the protests were raging. China has a history of protests when former leaders die—the 1998 Tiananmen Square crisis took place 3 months after Hu Yaobang’s death. And Jiang Zemin’s reforms brought China into the new era of WTO, 2008 Olympics, improving relations with the West, and explosive growth.
The FCC banned imports of telecom equipment from Huawei, ZTE, and surveillance equipment companies.
He was forced to relax Zero-COVID lockdowns and quarantine requirements without publicly admitting he was doing so.
Drowning in autocrats
There is no shortage of autocrats today. Based on Our World in Data figures, as of 2021, 70.7% of the world’s population (that’s 5.7 billion people) lived in countries ruled by autocrats (the red and orange areas in the graphic above), compared with just 46.4% in 2000. Examples include Vladimir Putin in Russia, Aleksandr Lukashenko in Belarus, Viktor Orbán in Hungary, Xi Jinping in China, Nicolás Maduro in Venezuela, and the stout young man with bad hair, whose missiles have killed more fish than any despot in history, in North Korea.
There are many reasons why autocracies are on the rise. In my last post, I wrote about The Real Long Covid as one factor today. After 3 years of pandemic, people are scared, demoralized, and have lost faith in institutions. Frightened people retreat into tribes where they support strongman leaders who promise to protect them against other tribes, backwards movements in societies known as the Savonarola Effect. Nowhere has that been more apparent than in US politics, where an angry mob stormed the Capitol building two years ago. In this post, however, I want to discuss a different aspect of autocracy, why autocrats make it more difficult to invest.
Autocrats make investing difficult
There is an urban myth that I’ve heard from dozens of CEOs and investors over the years. Democracies and other systems where no single individual has the power to make all decisions, are slow, messy, and inefficient, they say; it’s easier to do business and make investments in countries run by a single leader with an iron hand. In one sense they are right. It is easier to make a deal with one guy than to fight your way through an army lawyers, accountants, and regulators. But that doesn’t mean doing business with autocrats achieves higher returns or lower risk. To the contrary, when things go wrong in an autocracy—for example when one autocrat is replaced by another—they all go wrong at once.
Systems, like market economies, where change is the result of many people making independent decisions are messy but inherently more stable over long periods of time. Autocracies are stable over short periods but can produce the sudden, unpredictable tail events that we call Black Swans.
The following quote from Ilya Prigogine’s The End of Certainty was taken from a report made by a team of physicists to the European Communities. (Progogine won the 1977 Nobel Prize in Chemistry for his work on self-organizing systems.
“The maintenance of organization in nature is not—and cannot be—achieved by central management; order can only be maintained by self-organization. Self-organizing systems allow adaptation to the prevailing environment, i.e., they react to changes in the environment with a thermodynamic response which makes the systems extraordinarily flexible and robust against perturbations against outside conditions.” p. 71.
This is, of course, is why Friedrich von Hayek believed market economies are more stable than centrally-planned economies.
Nice error terms and the Central Limit Theorem
Investors need to understand this because we have been taught otherwise. Economics and finance textbooks typically give a nod to uncertainty by showing an error term at the end of each behavioral equation. But they assume the error terms are “nice”, i.e., independently and identically distributed normal distributions with known means and variances.
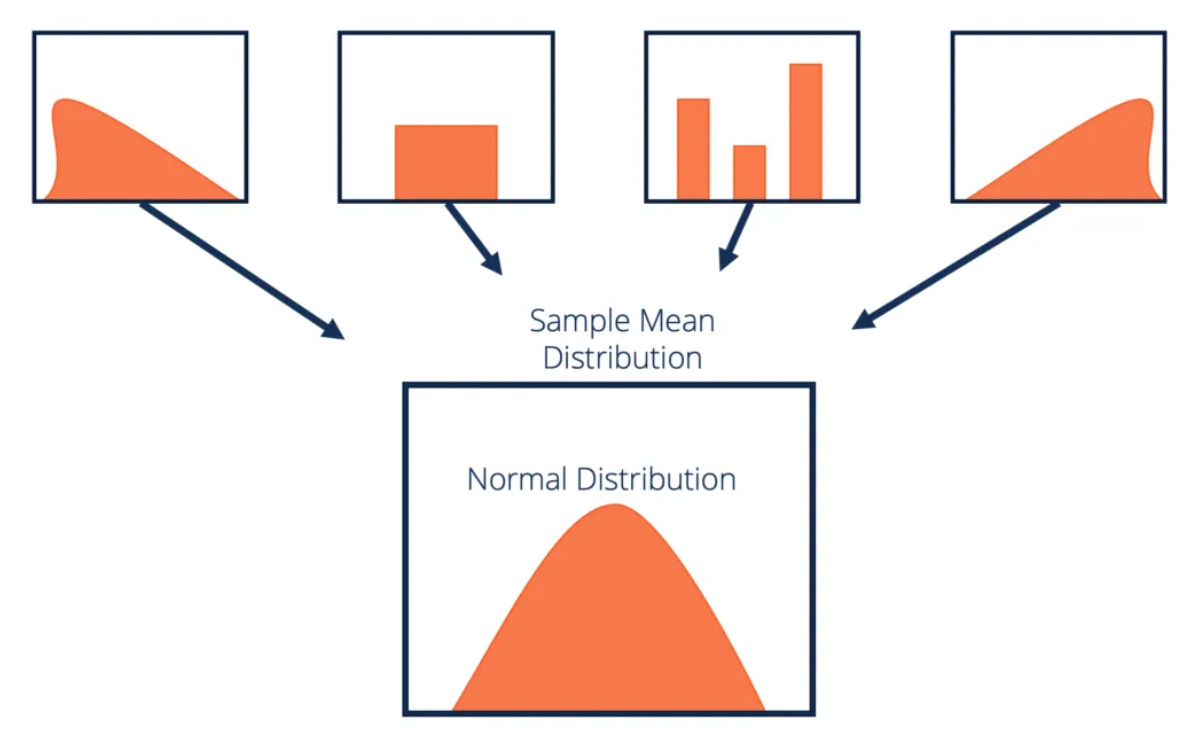
This assumption is often justified by invoking the Central Limit Theorem (CLT)—probability theory’s law of gravity—which states that the sample means (arithmetic averages) of a large number of independent observations will tend to be distributed normally even if the populations from which the samples were drawn were not normal. (cool, huh?).
[Note to readers: I am not 100% sure that I have the previous paragraph right. In my defense, I invoke the Central Limit Theorem (CLT), which says that even if I have it wrong, if I wrote the paragraph over again thousands of times, the average of those paragraphs would get it about right.]
You will of course, recognize the CLT from the market commentaries you read every day. They talk about “mean-returning” returns and valuation metrics (P/E, P/S, and P/FCF), and of “regressing toward the mean” and justify doing so with “the law of large numbers”. What they mean is if you see a big positive or negative change today, tomorrow’s change is likely to be closer to the hump in the middle of our old friend, the bell curve, i.e., the average from history.
There are two problems with this analysis. All are important for investors.
First, we don’t always have the “large number of observations” we need for the CLT to hold water. That’s especially true in macroeconomics work using scant quarterly data. The CLT is not a fact; it is a tendency that holds “in the limit”. Many otherwise well-educated people think that 30 observations is close enough to “in the limit” for government work. (The Corporate Finance Institute website, for example, states “the sample size must be 30 or higher for the central limit theorem to hold.” Nonsense. I am reasonably comfortable that the number 30 was made up by some guy who wanted to get a paper published and only had 31 data points.)
Second, the observations may not be independent, certainly not in the case of decisions made by an autocrat. A decision made by an autocrat on Tuesday might be statistically related to a decision made by the same guy on Monday.
Autocracies fail both tests. There is only one guy making decisions, the same guy for every decision, which may be why autocracies, in history, are associated with large outlier events—coups, revolutions, and wars. In practice, that means an autocrat with a garage full of nuclear weapons could wake up in a bad mood, or fall down the stairs on his way to breakfast, and do something rash that makes a lot of investment decision look bad all at once.
The two most prominent examples of autocracy risk today are Vladimir Putin’s repeated threats to use nuclear weapons and Xi Jinping’s not-so-subtle hints that he might use military force to seize Taiwan. Both make rational planning impossible, which is why I repeatedly advise investors to stay away from both China and Russia.
In fairness, I have not seen an empirical study that measures the relationship between power law exponents (black swan risk) and the effective number of people making decisions in democratic vs autocratic structures. I think that would be a valuable piece of research.
The real world, of course, is more complicated than my example. There are no pure democracies any more. And systems that appear autocratic—for example, tribal cultures and parliamentary monarchies—can have structures that ensure many people have been consulted before major decisions are made.
For example, during the time of Hu Jintao, no major decision would have been announced that had not first been circulated and discussed by the hundred or so CCP Politburo Standing Committee members and other senior leaders whose official residences are in Zhongnanhai, across the street from the Great Hall of the People. (They literally have red phones on their desk.) Since the rise of Xi Jinping’s power, this tradition has withered away. Most countries are somewhere between my two extremes.
As always, I welcome your comments.
Dr. John
APPENDIX: Power Laws
There may be a third reason why autocrats are associated with extreme events. I would conjecture that autocratic systems are more prone to extreme events—have longer tails—than less centralized forms of government. If so, autocratic behavior should have a predictable impact on estimates of the statistical properties of financial variables.
Financial variables, like rates of return, drawdowns, and changes in commodity prices and exchange rates are known for being, in the words of Igor in Young Frankenstein, “Abbe-Normal”. In particular, their distributions tend to resemble Power Law distributions, which are known for having fat tails and producing extreme outliers. (For good, readable explanations of Power Law distributions check out these books by Bak, Montier, and Sornette. I use them all in my Far-From-Equilibrium Finance class.)
Power laws are known for having huge, fat tails, i.e., for having a lot of shockingly big (or small) data points. They are expressed as “x to the kth power”—sorry but substack doesn’t have superscripts.
Power Law distributions slope sharply downward, like ski slopes. Higher values of k mean steeper slopes: k>1 are beginner’s slopes; k>2 is for intermediate skiers; and k>3 is for thrill-seeking, advanced skiers only. The fact that Power Law distributions only have one parameter (k) makes it unlikely they will have both a mean and a variance, CLT requirements. In fact, the mean of a Power Law is only defined for really steep ones, (k>2), and their variance is only finite for the black-diamond runs, (k>3).
Power Laws are ubiquitous in both natural and social phenomena, as shown in Bak. Examples include the Richter scale (actually the Gutenberg-Richter Law) measuring the intensity of earthquakes, Mandelbrot’s work on the prices of stocks, cotton, and other commodities, Raup’s “kill curve” on the distribution of species-extinction events over 650 million years, Zipf’s Law describing the distribution of population-density of the world’s cities, and the frequency of word-use in the English language, and Bak’s work on avalanches.
Most estimates of real-world power laws have exponents in the midrange (2<k<3), the area where a Power Law distribution has a well-defined mean butt does not have a finite variance, which means they display black swan behavior. For these distributions, as with California earthquakes, the next one could always be the big one.
Based on my reading of financial history, I would conjecture that power laws for financial metrics in systems controlled by autocratic regimes, should reveal much higher variances (smaller Power law exponents and longer tails) than similar metrics made in systems characterized by shared decision-making. If this were true, it could help us understand the relative performance of developed and less-developed countries. In fairness, I have not seen an empirical study that measures the relationship between power law exponents (black swan risk) and the effective number of people making decisions in democratic vs autocratic structures. I think that would be a valuable research project.
Dr. Rutledge, thank you for the great piece. I could read/talk about power laws all day. I think the theory that autocratic regimes increase power law behavior is a great one. I would imagine that if we look at the data, markets under autocratic regimes will experience a high number of small variations and a small number of extreme variations. Autocrats don't like volatility so natural evolution gets squashed out and they're left with a system lacking enough medium (healthy) volatility to clear out the garbage. Everything looks great until cleaning day happens all at once. If anyone picks up this thread for a research project, here are a few good resources on the empirics of power laws that I found very helpful when writing one of my papers. https://arxiv.org/pdf/0706.1062.pdf & https://arxiv.org/pdf/2001.10488.pdf